Business intelligence in insurance industry is revolutionizing how insurers operate, transforming data into actionable insights. From claims processing to risk assessment, BI empowers insurance companies to make data-driven decisions, optimizing operations, and enhancing customer experiences. This article delves into the core concepts, applications, and future of BI in the insurance sector, exploring various aspects like data sources, analytics, and regulatory compliance.
This comprehensive overview will cover a range of critical topics, from the fundamentals of business intelligence in the context of insurance to the advanced applications of data analytics for risk management and customer relationship management. We’ll also examine emerging trends and challenges in implementing and managing BI systems within the insurance industry. The aim is to equip readers with a deeper understanding of how BI is reshaping the insurance landscape.
Introduction to Business Intelligence in Insurance: Business Intelligence In Insurance Industry
Business intelligence (BI) in the insurance industry encompasses the use of data analysis techniques to gain insights into customer behavior, market trends, and operational efficiency. This allows insurance companies to make informed decisions, optimize pricing strategies, enhance customer service, and ultimately improve profitability. By leveraging data-driven insights, insurance companies can adapt to evolving market demands and maintain a competitive edge.
Key Characteristics of Insurance BI Systems
Insurance BI systems are specifically designed to handle the unique complexities of the insurance industry. They typically feature robust data warehousing capabilities, allowing for the storage and management of vast amounts of policy data, claims information, and customer demographics. Advanced analytics tools are incorporated to uncover patterns and correlations within this data. These systems often include sophisticated reporting and visualization tools, enabling stakeholders to easily interpret complex information and derive actionable insights.
Furthermore, security and compliance features are crucial to protect sensitive customer data and adhere to regulatory requirements.
Data-Driven Decision-Making in Insurance
Data-driven decision-making is essential for insurance companies to thrive in today’s competitive landscape. By leveraging historical data and market trends, insurance companies can make more accurate predictions about future claims, assess risk more effectively, and set appropriate premiums. This approach leads to more efficient resource allocation and improved operational performance. Real-world examples include insurers using predictive modeling to identify high-risk drivers and adjust premiums accordingly, or using customer segmentation to tailor marketing campaigns and enhance customer satisfaction.
Benefits of Implementing BI in Insurance Operations
Implementing BI in insurance operations yields a multitude of benefits. Improved risk assessment leads to more accurate pricing models, reducing the risk of financial losses. Enhanced customer understanding allows for the development of personalized products and services, increasing customer satisfaction and loyalty. Operational efficiency is also boosted through the identification of bottlenecks and inefficiencies in processes. Predictive analytics enables proactive risk management, enabling insurers to anticipate and mitigate potential issues before they escalate.
Types of Insurance Products and BI Application
Insurance companies can leverage BI to gain valuable insights into various insurance product types. A comprehensive understanding of these insights allows them to tailor their strategies for maximum effectiveness.
Insurance Product Type | BI Application Examples |
---|---|
Life Insurance | Analyzing mortality rates and identifying trends to refine pricing models, segmenting customers based on risk profiles to offer personalized products, and detecting fraudulent activities. |
Health Insurance | Predicting future claims costs based on historical data and demographics to adjust premiums and optimize resource allocation, identifying high-cost procedures to offer preventive care and cost-effective solutions, and enhancing customer experience with personalized care plans and proactive health recommendations. |
Auto Insurance | Analyzing accident patterns and driver behavior to identify high-risk drivers and adjust premiums accordingly, predicting future claim frequency and severity to optimize pricing and resource allocation, and developing targeted safety campaigns based on identified risky driving behaviors. |
Data Sources and Collection
Business intelligence (BI) in the insurance industry relies heavily on a vast array of data points. Understanding these sources and how they are collected, integrated, and managed is crucial for deriving actionable insights. Effective BI systems leverage this data to optimize pricing, improve risk assessment, and enhance customer service.
Data Sources
The insurance industry gathers data from diverse sources, each providing unique insights. Key data sources include claims data, policy data, market trends, demographic information, and external factors like economic indicators. Claims data, for example, reveals patterns in injury types, locations, and claim amounts. Policy data provides details on insured individuals and assets, allowing for the assessment of risk profiles.
Market trends encompass factors like inflation, interest rates, and the overall health of the economy, influencing pricing models.
Data Collection Methods
Collecting data from these varied sources requires sophisticated methods. Insurance companies often employ automated systems to collect and process claims data, which is frequently stored in structured formats. Policy data, which may reside in disparate systems, needs careful integration and standardization. Market trend data is typically sourced from external databases and financial reports. The collection process needs to be efficient and secure to prevent data breaches and ensure data integrity.
Data Integration Challenges
Integrating data from multiple sources presents several challenges. Data formats may differ significantly across various systems, requiring significant transformation efforts. Data quality issues, such as inconsistencies and missing values, can compromise the accuracy of analyses. Maintaining data consistency and ensuring compatibility between different systems requires careful planning and execution. Furthermore, ensuring data security throughout the entire process is critical to protect sensitive customer information.
Data Quality Management System
A robust data quality management system is essential for ensuring the accuracy and reliability of insurance BI. This system should encompass several key elements: data validation rules, data cleansing procedures, and regular data audits. Data validation rules should be implemented to identify and correct errors early in the data pipeline. Data cleansing procedures should be used to address inconsistencies and missing values.
Regular data audits are crucial for maintaining data quality and identifying potential issues.
Data Formats and Processing
- Structured Data: This data is organized in a predefined format, such as tables or databases. Examples include policy details, claims information, and customer demographics. Structured data is typically processed using SQL queries or relational database management systems (RDBMS) for analysis.
- Unstructured Data: This data lacks a predefined format and includes documents, images, and videos. Insurance companies may use this type of data, such as customer feedback or accident reports, to understand customer sentiment or identify potential fraud patterns. Processing unstructured data requires specialized tools and techniques, like natural language processing (NLP) or image recognition.
- Semi-structured Data: This data has some organizational structure, but not as rigid as structured data. Examples include XML or JSON files. These files can be parsed and used in BI systems for analysis.
Data Format | Description | Processing for BI |
---|---|---|
CSV (Comma Separated Values) | Plain text format, widely used for data exchange | Can be imported into spreadsheets or databases for analysis |
JSON (JavaScript Object Notation) | Lightweight format for data interchange, commonly used in APIs | Can be parsed and used in BI tools for data visualization and reporting |
XML (Extensible Markup Language) | Markup language for encoding documents, often used for configuration or metadata | Can be parsed and used in BI tools for data extraction and transformation |
Data Warehousing and Modeling
A robust data warehouse is crucial for effective Business Intelligence (BI) in the insurance industry. It acts as a central repository for all relevant data, enabling sophisticated analysis and reporting. This centralized storage facilitates a unified view of the insurance landscape, allowing for a comprehensive understanding of customer behavior, claims patterns, and market trends.Data warehousing in insurance BI involves the systematic collection, transformation, and loading of data from various sources into a structured environment.
This structured data allows for efficient querying and reporting, providing actionable insights for informed decision-making. The insights gained can be used to optimize pricing strategies, improve risk assessment, and enhance customer service.
Architecture of a Data Warehouse for Insurance BI
A data warehouse for insurance BI typically follows a three-tiered architecture. The first tier involves the source systems, encompassing claims data, policy data, customer data, and market data. The second tier comprises the ETL (Extract, Transform, Load) process, which transforms and loads data from source systems into the warehouse. The final tier is the data warehouse itself, housing the integrated and cleansed data in a structured format optimized for analysis.
This architecture enables efficient querying and reporting, allowing analysts to derive valuable insights.
Data Transformation and Loading Processes
Data transformation is a critical step in creating a consistent and usable data warehouse. This involves cleaning, standardizing, and enriching the data from various source systems. Data loading processes ensure the efficient transfer of transformed data into the warehouse. Different methods, such as batch processing and real-time loading, are employed depending on the volume and frequency of data updates.
This process ensures data integrity and consistency. For example, a batch process might be used for nightly updates, while real-time loading might be implemented for high-frequency data such as fraud detection alerts.
Importance of Data Modeling Techniques in Insurance BI
Data modeling is essential for structuring the data in the warehouse. Different modeling techniques cater to various analysis requirements. These models allow for efficient querying, reporting, and data mining. By structuring the data warehouse using appropriate models, analysts can quickly extract the required information. Properly designed models support accurate data analysis and forecasting, enabling better risk assessment and pricing strategies.
Role of Data Mining Techniques in Identifying Patterns and Trends
Data mining techniques are used to discover hidden patterns and trends in insurance data. Techniques such as association rule mining can identify correlations between policy features and claim frequencies. Clustering techniques can group similar customers based on their behavior, allowing for targeted marketing and risk assessment. Classification techniques can predict future claims based on historical data. These techniques enable a deeper understanding of insurance risks, leading to improved pricing and policy design.
Data Modeling Techniques for Insurance Industry Use Cases
Data Modeling Technique | Description | Use Case in Insurance |
---|---|---|
Star Schema | A simple model with a central fact table and multiple dimension tables. | Tracking policy sales, claim amounts, and customer demographics. |
Snowflake Schema | An extension of the star schema, with more detailed dimension tables. | Analyzing complex relationships between policies, customers, and claims, including location and other specific details. |
Entity-Relationship (ER) Model | A conceptual model that defines entities and relationships between them. | Defining the structure of the data warehouse, including entities like policies, customers, and claims, and their relationships. |
Dimensional Modeling | An approach to modeling data for analysis and reporting. | Developing reports on customer behavior, claim patterns, and profitability. |
BI Tools and Technologies
Business intelligence (BI) tools are crucial for insurance companies to analyze vast amounts of data and gain valuable insights. These tools empower companies to make data-driven decisions, optimize operations, and enhance customer service. Selecting the right BI platform is vital for achieving these goals.
Available BI Tools and Technologies for Insurance
A variety of BI tools are available, each with its strengths and weaknesses. Popular choices include cloud-based platforms, on-premise solutions, and open-source options. The best choice depends on the specific needs and resources of the insurance company. Factors to consider include data volume, existing IT infrastructure, and budget.
Unlocking the potential of business intelligence in the insurance industry requires a deep understanding of customer needs. Just as finding the perfect halal meal requires knowing your preferences, navigating the intricacies of risk assessment and policy optimization demands a similar level of insightful data analysis. For a quick lunch, discovering delicious halal restaurants near me now halal restaurants near me now is key.
Ultimately, leveraging these insights can lead to unparalleled profitability and customer satisfaction in the insurance sector.
Comparison of BI Platforms for Insurance
Different BI platforms offer varying functionalities and capabilities. Cloud-based platforms often provide greater scalability and flexibility, while on-premise solutions offer more control over data security and infrastructure. Open-source tools can be cost-effective but may require more technical expertise. Each platform’s strengths and weaknesses must be carefully weighed against the insurance company’s specific requirements.
Key Features and Functionalities of Leading BI Tools
Leading BI tools often share core functionalities such as data visualization, reporting, and data mining. Sophisticated tools may also include advanced predictive modeling capabilities to forecast future trends and identify potential risks. These capabilities are critical for insurers to assess customer behavior, understand claims patterns, and improve risk management. Examples include features for interactive dashboards, real-time data analysis, and automated reporting.
Integration of BI Tools with Existing Insurance Systems
Successful integration of BI tools with existing insurance systems is essential for seamless data flow and efficient analysis. This integration process can be complex, involving data migration, API development, and system configuration. Choosing tools with robust APIs and well-documented integration guides will ease this process.
Table: Pros and Cons of Different BI Tools
BI Tool | Pros | Cons | Pricing | Scalability | Integration |
---|---|---|---|---|---|
Tableau | Excellent visualization capabilities, user-friendly interface, strong community support. | Can be expensive, limited in-depth data mining capabilities compared to specialized tools. | Generally, a tiered pricing structure based on features and user count. | Highly scalable, with cloud options for larger datasets. | Good integration capabilities through APIs and connectors. |
Power BI | Strong integration with Microsoft ecosystem, affordable for smaller companies, user-friendly. | May not be as powerful for complex analytics as specialized tools, limited customization options. | Offers various pricing tiers, including free options. | Scalable with cloud-based solutions. | Integrates well with other Microsoft products. |
Qlik Sense | Excellent for data discovery and exploration, rich visualization features, strong scalability. | Steeper learning curve compared to Tableau or Power BI, potential licensing costs. | Usually a subscription-based model, with various pricing options. | Highly scalable, capable of handling large datasets. | Provides robust APIs and integration capabilities. |
Analytics and Reporting
Insurance businesses leverage Business Intelligence (BI) to gain actionable insights from their vast datasets. This allows them to make data-driven decisions, optimize operations, and enhance customer experiences. A crucial aspect of BI in insurance is the application of analytical techniques and the creation of reports and dashboards to extract meaningful information from the collected data.Effective BI implementation in insurance goes beyond simply gathering data; it requires transforming raw data into valuable knowledge.
This is achieved through sophisticated analytical approaches and well-designed visualizations, ultimately supporting informed decision-making in areas like pricing, risk assessment, and claims management.
Types of Analytics in Insurance BI
Insurance companies employ various analytical techniques to extract value from their data. These techniques range from simple descriptive analysis to more complex predictive and prescriptive approaches. Understanding these different types of analysis is crucial for extracting actionable insights.
- Descriptive Analytics: This involves summarizing historical data to understand past performance. For example, analyzing claim frequency over the past five years can reveal trends and patterns in specific geographical regions or customer segments. This insight can then inform proactive risk management strategies.
- Predictive Analytics: This uses statistical modeling to forecast future outcomes. For instance, predictive models can predict the likelihood of a customer churning based on factors such as age, policy type, and claim history. This enables proactive customer retention strategies.
- Prescriptive Analytics: This goes beyond prediction by recommending actions to optimize outcomes. For example, prescriptive analytics can suggest optimal pricing strategies based on predicted customer behavior and market conditions, potentially maximizing profitability while maintaining competitiveness.
Generating Insightful Reports, Business intelligence in insurance industry
Effective reports are crucial for translating BI insights into actionable strategies. These reports should be tailored to the specific needs of different stakeholders within the insurance organization. A robust reporting framework ensures consistent and reliable information dissemination.
- Report Structure: Reports should be structured logically, with clear headings, tables, and visualizations. Key performance indicators (KPIs) should be prominently displayed. The format should be easily understandable for the intended audience.
- Content Focus: Reports should highlight key trends, patterns, and anomalies. Presenting the data in a concise and impactful way will maximize comprehension and foster informed decision-making.
Creating Dashboards and Visualizations
Dashboards and visualizations are critical for effectively communicating BI insights to different stakeholders. Interactive dashboards allow users to drill down into data and explore different perspectives.
- Dashboard Design: Dashboards should be designed with user experience in mind, featuring intuitive navigation and easily digestible information. Visualizations should be clear, concise, and relevant to the specific stakeholders’ needs.
- Visual Storytelling: Visualizations should tell a story about the data. Using a variety of charts and graphs, dashboards can highlight key trends, patterns, and insights. A well-designed dashboard can reveal important trends in claim frequency, customer churn, and policy profitability.
Role of Visualization in Conveying Insights
Visualizations play a crucial role in conveying complex data in a readily understandable format. Visual representations help in identifying patterns, anomalies, and insights that might otherwise remain hidden in raw data. This makes data-driven decision-making more effective.
- Choosing Appropriate Visualizations: The choice of visualization (e.g., bar charts, line graphs, pie charts, scatter plots) should be based on the type of data and the intended message. For example, a bar chart is suitable for comparing claim frequency across different regions, while a scatter plot might be more appropriate for analyzing the relationship between policy premiums and customer demographics.
Examples of Visualizations for Insurance Aspects
Insurance Aspect | Visualization Type | Description |
---|---|---|
Claim Frequency | Line Graph | Displaying claim frequency trends over time, highlighting seasonal patterns or sudden spikes. |
Customer Churn | Pie Chart | Illustrating the percentage of customers who churned in different customer segments. |
Policy Profitability | Bar Chart | Comparing the profitability of different policy types, highlighting high-performing and low-performing ones. |
Risk Assessment | Heatmap | Visualizing the risk levels associated with different customer segments or geographical locations. |
Risk Management and Fraud Detection
Business intelligence (BI) is increasingly crucial in the insurance industry for managing risks and detecting fraud. Leveraging data analysis, BI empowers insurers to make informed decisions, optimize operations, and enhance profitability. This is achieved by identifying potential risks, assessing their impact, and implementing strategies to mitigate them. It also allows for proactive fraud detection, reducing financial losses and maintaining trust with policyholders.The insurance industry is highly susceptible to various risks, including policyholder fraud, natural disasters, and economic downturns.
BI systems can analyze vast datasets to identify patterns and anomalies, enabling proactive risk management strategies. By employing advanced analytical techniques, insurers can predict potential losses and adjust their pricing and coverage accordingly. The use of BI in fraud detection is critical, helping to identify suspicious claims, minimize financial losses, and preserve the integrity of the insurance system.
Identifying and Mitigating Risks
BI enables insurers to identify and quantify various risks affecting their portfolios. Through historical data analysis, insurers can recognize trends, predict future events, and adjust their strategies to mitigate risks. By analyzing claims data, for example, insurers can pinpoint geographical areas with higher claim frequencies or types of policies with elevated loss ratios. These insights allow insurers to adjust pricing, modify coverage terms, or invest in preventive measures to reduce future losses.
Furthermore, BI can analyze market trends, economic indicators, and competitor activities to identify potential external risks.
Role of BI in Fraud Detection and Prevention
BI plays a pivotal role in detecting and preventing insurance fraud. Sophisticated algorithms can analyze claim data, policyholder information, and other relevant data points to identify unusual patterns and suspicious activities. These algorithms can detect fraudulent claims based on inconsistencies in reporting, suspicious correlations between multiple claims, or unusual patterns in claim frequency or severity. For example, a sudden surge in claims from a particular region for a specific policy type might trigger an investigation.
This allows insurers to quickly respond to fraudulent activities and take preventive measures to reduce future instances.
Assessing and Managing Insurance Portfolios
BI provides a powerful framework for assessing and managing insurance portfolios. Insurers can use BI tools to segment their portfolios based on various factors, such as demographics, risk profiles, and claim history. By understanding the characteristics of different policyholder groups, insurers can tailor their products, pricing, and marketing strategies to better meet their needs. Further, insurers can identify potential weaknesses within their portfolios and implement strategies to strengthen them.
For example, if a specific type of policy is proving unprofitable, BI can reveal the reasons behind the losses and help develop strategies to address the issue.
Developing Risk Assessment Models using BI
Developing robust risk assessment models is a crucial application of BI in the insurance industry. The process typically involves collecting relevant data, defining risk factors, building predictive models, validating model accuracy, and deploying the models for risk assessment and decision-making. A crucial aspect of model development is data preparation. Data needs to be cleansed, transformed, and modeled to ensure its accuracy and suitability for predictive analysis.
Statistical techniques, such as regression analysis and machine learning algorithms, can be employed to build models that predict the likelihood of claims or losses based on various risk factors.
Risk Management Strategies Aided by BI
Risk Management Strategy | Description | BI Application |
---|---|---|
Pricing Adjustments | Adapting premiums based on risk factors. | Analyzing historical claims data to identify correlations between risk factors and claim frequency/severity. |
Policy Modifications | Adjusting policy terms and conditions to mitigate specific risks. | Identifying policy types with high loss ratios to adjust coverage or exclusions. |
Preventive Measures | Implementing measures to reduce the likelihood of risks. | Identifying geographic areas with high claim frequency to invest in safety programs. |
Fraud Detection and Prevention | Implementing measures to reduce fraudulent activities. | Developing algorithms to identify unusual patterns in claims data and policyholder behavior. |
Customer Relationship Management (CRM) and BI
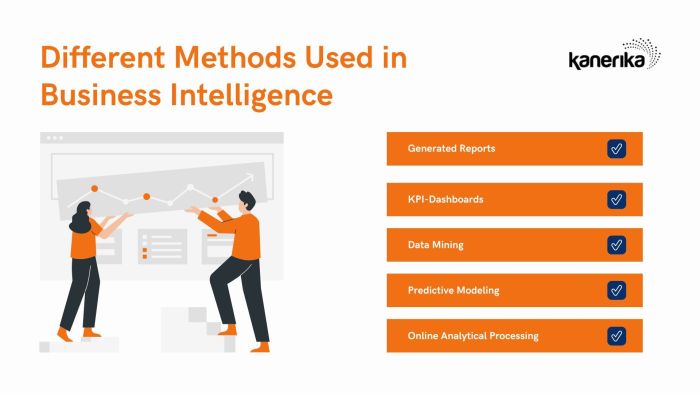
Customer relationship management (CRM) systems are critical in the insurance industry for building strong customer relationships and driving sales. Business intelligence (BI) tools can significantly enhance these efforts by providing valuable insights into customer behavior and preferences. This allows insurance companies to personalize offerings and tailor their services to individual customer needs, leading to increased customer satisfaction and loyalty.BI plays a pivotal role in understanding customer needs and preferences.
By analyzing data from various sources, BI tools can identify trends, patterns, and correlations that might otherwise be missed. This understanding allows insurers to develop more effective CRM strategies and create targeted campaigns that resonate with specific customer segments.
Relationship Between CRM and BI
CRM systems focus on managing customer interactions, while BI systems focus on analyzing data to gain insights. Combining these two allows insurers to create a holistic view of their customers. BI data can inform CRM strategies, enabling more personalized and effective interactions. For instance, understanding customer buying habits through BI can lead to more targeted marketing campaigns within the CRM system.
BI Enhancing Customer Relationship Management
BI tools can provide valuable insights for improving customer service and retention. Analyzing customer service interactions, such as call logs and email correspondence, allows insurers to identify areas for improvement in their processes. This data-driven approach to customer service leads to a more efficient and responsive service experience, ultimately strengthening customer relationships.
Personalized Insurance Offerings
BI enables the creation of highly personalized insurance offerings. By analyzing customer data, including demographics, risk profiles, and buying habits, insurers can tailor insurance products to meet specific needs. This personalized approach can lead to increased customer satisfaction and higher conversion rates. For example, a young, healthy driver might receive a lower premium than an older, less experienced driver.
Customer Segmentation in Insurance BI
Customer segmentation is crucial for effective BI in insurance. By dividing customers into distinct groups based on shared characteristics, insurers can tailor their marketing strategies and products. Different customer segments might respond differently to various communication channels or product features. For instance, a segment focused on families might respond favorably to bundled insurance products, while a segment of business owners might be more interested in tailored commercial packages.
CRM Strategies vs. BI Implementations for Customer Service
CRM Strategy | BI Implementation for Customer Service |
---|---|
Reactive customer service, responding to complaints and requests | Proactive customer service, identifying potential issues before they escalate using predictive modeling and historical data analysis. |
Generic support channels (e.g., phone, email) | Personalized support channels (e.g., chatbots, targeted emails, personalized FAQs) |
Limited data collection and analysis on customer interactions | Comprehensive data collection and analysis on customer interactions, allowing for deeper understanding of customer needs and pain points. |
Focus on immediate issue resolution | Focus on long-term relationship management by identifying patterns and trends in customer behavior and anticipating future needs. |
Regulatory Compliance and BI
Insurance companies face a complex web of regulations, and maintaining compliance is crucial for their long-term success. Business intelligence (BI) plays a vital role in navigating these requirements by providing a structured approach to data analysis and reporting. This allows insurers to identify potential risks, proactively address compliance issues, and ultimately reduce the risk of penalties and reputational damage.BI empowers insurers to efficiently manage the intricate data needed to demonstrate regulatory adherence.
This includes not only collecting and analyzing data, but also securely storing and accessing it to satisfy regulatory inquiries. Effective BI implementation directly contributes to streamlined compliance processes and fosters a culture of accountability within the organization.
Regulatory Compliance Reporting
Effective compliance reporting is paramount for insurance companies. BI tools provide a platform for generating reports that meet specific regulatory requirements, such as those Artikeld by the relevant insurance authorities. These reports can cover various aspects, including policyholder data, financial performance, and risk assessments. Automated reporting processes, enabled by BI, drastically reduce the time and resources needed for manual compilation.
This efficiency translates to faster turnaround times and a more agile response to regulatory scrutiny.
Data Governance and Security in Insurance BI
Data governance and security are fundamental to any successful BI implementation in the insurance sector. Robust data governance frameworks ensure data quality, accuracy, and consistency. This includes establishing clear data ownership, access controls, and procedures for data validation. Data security protocols, crucial in protecting sensitive customer information, are integral to compliance with regulations like GDPR and others.
These protocols should safeguard against unauthorized access, data breaches, and ensure data confidentiality. The importance of data encryption and access restrictions cannot be overstated.
Ensuring Data Integrity and Compliance
Maintaining data integrity and compliance throughout the BI implementation process is essential. Implementing rigorous data validation procedures, coupled with regular audits, can significantly enhance data quality and reduce the risk of errors. This includes data cleansing procedures, which remove inconsistencies and inaccuracies, and ongoing monitoring to ensure data integrity. Furthermore, establishing clear procedures for data entry and updates will prevent issues and ensure compliance with regulatory guidelines.
Adherence to industry best practices for data security and access control is also critical.
Table of Regulatory Guidelines and BI Support
Regulatory Guideline | How BI Can Aid in Meeting the Guideline |
---|---|
Solvency II (EU) | BI can analyze financial data to assess solvency positions, model risks, and generate reports required by Solvency II regulations. This helps insurers meet the regulatory capital requirements. |
Insurance Regulatory and Development Authority (IRDAI) (India) | BI tools can be used to track and analyze insurance policies, premium collections, and claims settlements, facilitating compliance with IRDAI regulations. |
Insurance Act (Various Jurisdictions) | BI systems can track and report on policyholder data, financial transactions, and other relevant information to ensure adherence to the insurance act in various jurisdictions. |
Data Privacy Regulations (e.g., GDPR) | BI implementations should incorporate robust data privacy protocols, including data encryption, access controls, and data anonymization techniques, to ensure compliance with data privacy regulations. |
Future Trends and Challenges
The insurance industry is undergoing a rapid transformation, driven by technological advancements and evolving customer expectations. Business Intelligence (BI) plays a crucial role in navigating this shift, enabling insurers to adapt to new demands and capitalize on emerging opportunities. This section explores the key future trends and challenges facing insurance BI implementation.
Emerging Trends in Insurance BI
The insurance sector is witnessing a confluence of technological advancements that are reshaping how BI is used. These trends include the increasing use of cloud-based platforms for data storage and processing, the integration of Artificial Intelligence (AI) and Machine Learning (ML) models into BI systems, and a growing emphasis on real-time data analysis. Furthermore, the rise of the Internet of Things (IoT) is generating vast amounts of data that insurance companies can leverage to gain deeper insights into customer behavior and risk assessment.
Challenges of Implementing and Managing BI Systems
Implementing and managing robust BI systems in insurance presents a range of challenges. Data security and privacy are paramount concerns, particularly given the sensitive nature of customer information. Ensuring data accuracy and consistency across various sources is another significant hurdle. Furthermore, maintaining the system’s performance and scalability as the volume and velocity of data increase is critical.
Unveiling the intricate world of business intelligence in the insurance sector reveals a fascinating tapestry of data analysis. Understanding how insurance companies leverage this data is crucial to optimizing their operations. For instance, deciphering if a claim like bumper damage is covered by insurance, requires meticulous examination of policy terms, a task made easier by leveraging sophisticated business intelligence tools.
This insight, coupled with the ability to predict future trends and optimize risk management, is the cornerstone of success in the ever-evolving insurance industry. does insurance cover bumper damage Ultimately, business intelligence equips insurance providers to make informed decisions, strengthening their resilience and profitability in the market.
Finally, the need to adapt to evolving regulatory landscapes and compliance requirements poses a significant ongoing challenge.
Impact of Emerging Technologies on Insurance BI
AI and machine learning are transforming insurance BI by automating tasks, improving risk assessment, and enhancing customer service. For example, AI-powered chatbots can handle routine inquiries, freeing up human agents for more complex issues. Machine learning algorithms can identify patterns in claim data, allowing insurers to predict potential fraud or optimize pricing strategies. The increased availability of real-time data, coupled with the ability of AI and ML to process it, offers unparalleled opportunities for insurers to adapt to changing market conditions and customer demands.
Potential Future Applications of BI in Insurance
BI has the potential to revolutionize various aspects of the insurance industry. One potential application is in personalized risk assessment, enabling insurers to offer customized premiums based on individual risk profiles. Another is in fraud detection, where BI can identify anomalies and patterns indicative of fraudulent activities. Furthermore, predictive modeling can help insurers anticipate future claims trends and proactively adjust pricing strategies.
Table: Potential Impact of Future Technologies on Insurance Operations Using BI
Technology | Potential Impact on Insurance Operations (Using BI) |
---|---|
AI and Machine Learning | Improved risk assessment, fraud detection, personalized pricing, automated claims processing, and enhanced customer service. |
Cloud Computing | Increased scalability, reduced costs, improved data accessibility, and enhanced collaboration among teams. |
IoT | Improved risk assessment through real-time data collection, better understanding of customer behavior, and enhanced claims processing. |
Big Data Analytics | Improved insights into customer behavior and market trends, leading to better product development, pricing strategies, and customer relationship management. |
Ending Remarks
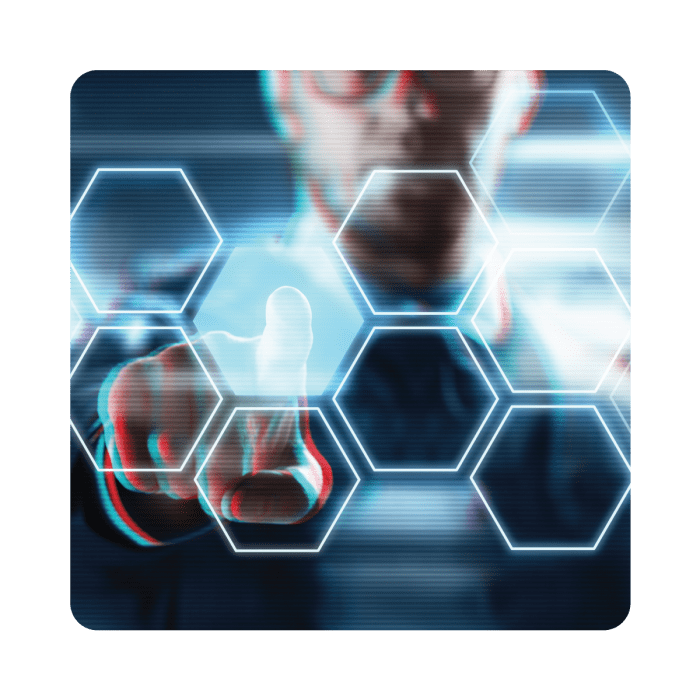
In conclusion, business intelligence is proving to be a powerful tool for insurance companies to gain a competitive edge. By leveraging data-driven insights, insurers can optimize their operations, enhance customer experiences, and mitigate risks. The future of insurance is intertwined with the ability to effectively utilize BI, and this article has highlighted the key aspects for success. From data collection and warehousing to advanced analytics and reporting, the discussed topics equip readers with a solid foundation to understand and navigate the complexities of BI in the insurance industry.
FAQ Insights
What are some common data sources used in insurance BI?
Common data sources include claims data, policy data, customer demographics, market trends, and external economic indicators. These sources provide a comprehensive view of the insurance landscape and allow for in-depth analysis.
How can BI help insurers manage risk?
BI enables insurers to identify potential risks more accurately, model different scenarios, and develop more effective risk mitigation strategies. This includes fraud detection, portfolio assessment, and pricing optimization.
What are the key challenges in implementing BI in insurance?
Challenges include integrating diverse data sources, ensuring data quality and accuracy, maintaining regulatory compliance, and keeping up with evolving technologies. These are significant hurdles that need careful consideration and planning.
How can BI enhance customer relationships in the insurance industry?
BI can personalize insurance offerings, segment customers effectively, and provide tailored support through data analysis. This ultimately leads to improved customer satisfaction and loyalty.